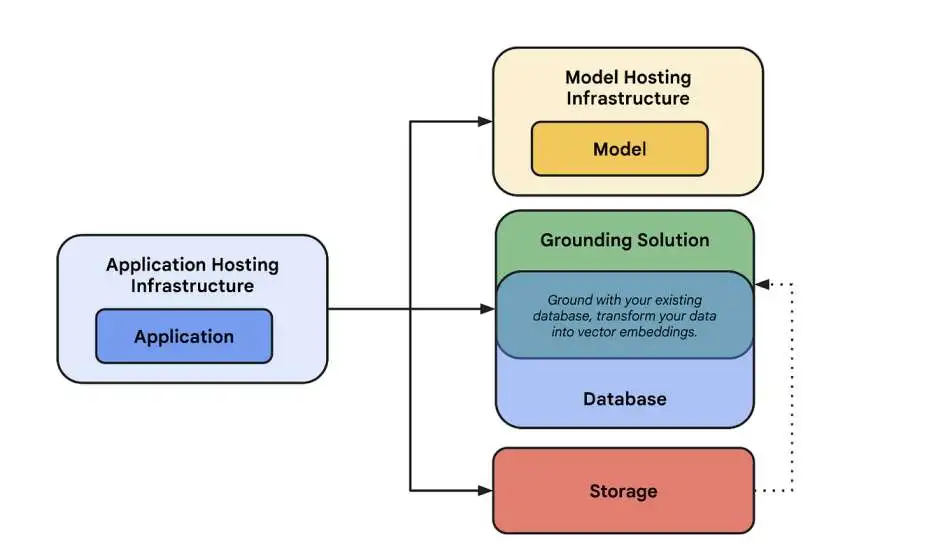
How Do I Access Llama-3 on Google Cloud?
Want to know how do i access llama-3 on google cloud? Whether you’re an ML expert or a newbie trying to tinker with the Meta Llama 3 version on your very own, Runhouse makes it smooth to leverage the compute resources you have already got (AWS, GCP, Azure, neighborhood gadget, etc.) and run Llama 3 with minimum setup.
In this manual, we’ll begin with a top level view of the Llama three version in addition to reasons for choosing an open LLM versus a proprietary model including ChatGPT. Then we’ll dive into an example on walking Llama 3 privately in your very own infrastructure the use of Runhouse.
Our principal instance uses Hugging Face Transformers and deploys to AWS but this newsletter gives extra code snippets for serving with TGI and vLLM. We’ll additionally cowl methods to set up to different cloud companies (GCP and Azure) and to your local gadget.
How Do I Access Llama-3 on Google Cloud?
Meta Llama 3
This past April, Meta introduced the state-of-the-art version of their open large language version (LLM), Llama 3. The new model has impressive benchmarks and has challenged the idea that proprietary fashions will continually offer superior overall performance in comparison to open ones.
Read Also: Why Isn’t Boolean Search Working On Google Talent Cloud 2024?
Before moving into the specifics of walking Llama three your self, we’ll cross over a brief evaluation of the model’s competencies and the tradeoffs between open and proprietary LLMs. We’ll additionally cover an overview of the to be had model variants. In this blog, to know complete guide on how cloud stratus c7 screenshot turn off google search bar?
Llama 3 Performance
The contemporary technology of Llama models from Meta demonstrates marvelous performance and has improved the range of opportunities with open LLMs.
The larger 70B model has even out-accomplished GPT-three.5 in Meta’s critiques. Meta has benchmarked the version against both open and proprietary models inclusive of Claud and Mistral. We encourage you to study greater of the information from Meta’s legitimate assertion.
Note that we’ll be the use of the smaller 8B parameter model on this manual. To host the 70B model, you can without difficulty replace our scripts to apply extra effective digital machines on your chosen cloud provider, however the cost is extensively higher.
Open v. Proprietary Models
You’ve likely attempted proprietary models including OpenAI’s ChatGPT, Anthropic’s Claude, or Google’s Gemini, which might be easily on hand with intuitive chat interfaces and nicely-documented APIs. These groups attention on handing over a effective tool that can manage a huge kind of uses.
The underlying models for each are absolutely non-public and might best be interacted with the usage of their APIs and tools. Of course, there are tradeoffs that come from closed, proprietary fashions, inclusive of:
Related Article: Google VPS Cloud: Transforming Your Digital Infrastructure
Data is despatched through a 3rd celebration API to engage with the version, main to privacy issues by doubtlessly sharing touchy statistics with a model hosting company.
The fee of inference is precisely managed by the version’s owner, allowing for very little manage over your spending.
Models may be updated at unpredictable intervals potentially produce inconsistent results, a subject for environments in which reproducibility is crucial.
Most proprietary fashions provide no way to first-rate-music the version to fit you, although some are experimenting with options in this space.
Especially for company use instances, issues associated with closed models may be a nonstarter. Businesses normally need extra manipulate over statistics protection, reproducible outcomes, observability, and alternatives for value optimization.
Open models such as Meta Llama 3 can be downloaded directly and used privately. This method which you don’t want to ship any statistics through a third birthday party API and you may host the model and run inference to your own infrastructure.
It’s greater prematurely paintings to deploy your version, and that’s something we’re fixing with Run residence. Scarcity and value of web hosting GPUs also can be a concern.
Pre-Trained and Instruction Tuned Models
You’ll word there are some distinctive Llama 3 fashions to be had for download on Hugging Face. The models are provided in sizes – 8B and 70B parameters – every in pre-trained and education tuned variants. The model size without delay impacts how plenty compute you’ll want to run inference.
If you’ve used ChatGPT and other AI tools, you’re acquainted with regular chat interfaces. However, on a simple level, LLMs work via predicting the maximum possibly text in a series with no special cognizance of questions or answers.
If you ran inference with a query at once to a pre-skilled version, the LLM would possibly respond with a listing of similar questions. By education a version to use a spark off format, it is able to produce solutions extra appropriate for a chat UI.
Llama 3 Instruct fashions are tuned to higher handle the prompting format, making them perfect for chat interfaces and anything concerning questions and solutions. We’ll use the smaller Llama three 8B Instruct model for our examples. Many tools, along with the Hugging Face transformers library (that we’ll use on this educational), include methods to apply chat templates.
How to Use Llama 3 with Run Residence
In this guide, we’ll describe how you could deploy a Llama 3 8B Instruct Model on AWS the use of Run residence. If you’d want to dive right into the code, please skip ahead to our instance tutorial. Trying to install on a distinct provider like Google Cloud Platform (GCP) or Azure? Be sure to skip in advance to the segment describing initialization of each in addition to advocated compute resources to set up.
Run residence is an open supply Python bundle that allows you to without difficulty expand, debug, and run code to your very own compute. Compatible with AWS, GCP, Azure, and extra, Runhouse is specially beneficial for speedy ML improvement by efficiently utilising your GPUs and CPUs. With a quick setup and some strains of Python code, you’ll be able to run inference on your own Llama 3 version.
Llama 3 Chat Module with Hugging Face Transformers
One of the most popular approaches to serve LLMs is with Hugging Face Transformers. This library gives an clean-to-use interface for operating with many open models together with Llama. Since we don’t take ourselves too critically, we’ll configure the version to reply like a pirate, but we encourage you to find a more (or far less) practical application for Llama three.
Deploy the far off Llama 3 Model
To start the usage of our HFChatModel we’ll need to run a script that sets up the module on a far flung cluster. The following sections will need to be run within a if __name__ == "__main__": block to make certain it isn’t run remotely by using Runhouse when the script is imported throughout deployment.
Serving Alternatives: TGI and vLLM
Depending to your man or woman use case, you may need to consider different alternatives for running inference on Llama three. Hugging Face Transformers is famous and clean to installation, but TGI (also from Hugging Face) and vLLM can function greater performant alternatives. Since Runhouse can set up any Python code in your digital machines, we can modify our Module to leverage those (or another) libraries.
Serving Llama 3 with TGI
For progressed performance, you could need to use Hugging Face’s TGI toolkit to serve the LLM rather than the popular transformers library from our example. TGI offers many optimizations that might enchantment for your manufacturing use instances.
We’ll define a new Runhouse Module that uses TGI to serve the same Llama three 8B Instruct version as the instance above. This Module will serve as a wrapper to install TGI as a Docker box on our cluster as a way to be wired as much as port 8080 to be easily accessed via HTTP requests or curl using the Messages API. Alternatively, you could bypass the open_ports and SSH into the cluster for progressed security.
Serving Llama 3 with vLLM
Alternatively, you may use vLLM to serve the Llama 3 version. VLLM is designed for higher throughput than both Transformers or TGI and can be a higher alternative for a high-call for software. There are nevertheless many tradeoffs which you must don't forget on your precise assignment and we encourage you to study thru vLLM’s documentation.
To implement vLLM in vicinity of Transformers, we’ll need a brand new Runhouse Module and script used to have interaction with the version. Below is a snippet from a category that hundreds a vLLM engine with Llama 3 and runs inference with a generate method. Note that this situation might want extra logic for a chat interface using activate templates.
For the entire, runnable Python document and extra facts, please go to our Meta Llama 3 with vLLM instance. This instance deploys to GCP and might serve as a helpful evaluation to see how Run house works with numerous cloud providers. What you want to know more guide on cloud stratus c7 screenshot turn off google search bar?
Run Llama 3 on Any Cloud or Locally
Run residence allows you to without problems deploy the identical module on any cloud infrastructure. All we need to do is update the Run house set up and make minor changes to the cluster definition in our deployment script. To make certain that you have the right credentials installation for every of these carriers, please run sky check to test earlier than trying to run the code.
Run Llama 3 on Google Cloud Platform (GCP)
Running on GCP is as easy as updating the Run house bundle installation and enhancing the definition of your cluster. First, re-import Run house with GCP and initialize your Google Cloud account. Next, replace the cluster definition to goal gcp because the company and L4:1 accelerators. This is a GPU model usually to be had on GCP and correctly sized for strolling the Llama three version.
Local Llama 3
There are few motives you may need to run Llama domestically. You may also sincerely need to run it for your computer for private offline usage, and not hassle with cloud VMs and accelerators.
For that, there are numerous fantastic alternatives imparting accelerated, out of the container support for lots commonplace private computing architectures, such as Ollama, llama.Cpp (overall performance-focused and written in C++) and LM Studio (consists of an easy-to-use UI).
Another not unusual use case for nearby execution is incorporating Llama three into an present utility like a FastAPI app. Running a single inference is simple but the reminiscence, latency, and concurrency issues of serving an software like this can be difficult. Need to know how do i access llama-3 on google cloud?
You need to make certain that calls to the model are multiplexed to take advantage of paging structures like vLLM, which can be a long way extra green than servicing calls one at a time. You also might also want to take benefit of replication through creating a couple of employees for your common app however don’t need to duplicate the model itself oftentimes in reminiscence (or restart vLLM with each replica).
Runhouse can send a vLLM module into a separate process shared by means of your replicas the usage of the rh.Right here approach.
This is a extraordinary easy way to make sure green multiplexing, clear concurrency handoffs, and no memory duplication. Assuming we’re on a GPU container, we can reuse our vLLM LlamaModel above as-is (and if now not, we will use transformers or a number of the neighborhood options above):